Data-driven high friction surface treatment applications for roadway safety
Handling editor: Reginald Souleyrette, University of Kentucky, the United States of America
Reviewers: Craig Lyon, Traffic Injury Research Foundation, the United States of America
Lindsay Walker, HMB Professional Engineers, the United States of America
Received: 14 June 2024; Accepted: 2 December 2024; Published: 7 January 2025
Abstract
The Kentucky Transportation Cabinet (KYTC) Highway Safety Improvement Program (HSIP) has been utilizing a data-driven high friction surface treatment (HFST) program as a proven safety countermeasure for wet weather and roadway departure crashes. This report will walk through the origins of the study and the process to identify candidate locations for HFST and development of a pavement friction program. The project has constructed over forty sites in 2023, including a mixture of rural corridor curves, signalized intersection approaches, and limited access freeway ramps. The project utilized a mixture of pavement friction data, pavement resurfacing lists, and specific crash data. The sites are being reviewed to show the benefits through a before and after crash analysis and pavement condition over time based on traffic volumes, weather, and other factors.
Keywords
high friction surface treatment, pavement friction data, wet crash prevention
Introduction
High friction surface treatment (HFST) has been applied across the Commonwealth of Kentucky for over ten years as a proven safety countermeasure as part of a reactionary approach to preventing and/or reducing wet roadway condition crashes. In 2020, the Kentucky Transportation Cabinet (KYTC) Highway Safety Improvement Program (HSIP) began developing a data-driven pavement friction program to holistically solve pavement friction issues and amplify traffic safety efforts. The program initially started using crash data as the source for identifying locations where pavement friction may be the source of wet roadway condition and/or roadway departure crashes. As crash data is a strong indicator of a problem, it does not tell the full story of whether pavement friction is the source of the problem. Thus, WDM USA was contracted to proactively collect pavement friction data to provide further data-driven detail to identifying locations where modifying pavement friction could assist in preventing an/or reduction wet roadway condition crashes.
In 2023, the WSP team was assigned a contract to work with KYTC HSIP, WDM USA, and the Kentucky Transportation Center (KTC) to utilize the current data and methods and begin identifying locations for HFST applications immediately. Two rounds of data analysis and field visits were performed in 2023 to identify and construct over forty sites in 2023, including a mixture of rural corridor curves, signalized intersection approaches, and limited access freeway ramps. Another round of HFST locations were identified in 2024 and will be constructed throughout the year during the construction season. All sites constructed are being reviewed to show the benefits through a before and after crash analysis, while also reviewing other factors such as pavement condition over time based on traffic volumes, weather, and other factors. Throughout the program, other assets and pavement texturing methods are being analyzed for maximizing KYTC's return on investment and assisting with the goal of preventing fatal and serious injury crashes on Kentucky roadways.
Methodology
The methodology for this pavement friction program follows a top-down approach centered primarily around data and utilizes field reviews for confirmation. The approach starts with data collection and combination, adding in supplemental data for screening prioritization, identifying locations for project grouping where applicable, develop candidate sites through a desktop review, conduct field reviews for site confirmation, and refine site bounds with project team and finalize for implementation. Figure 1 shows the process flow.

Data collection and combining
Multiple datasets and repositories were utilized to accurately predict locations best suited for HFST. The datasets provided and collected by KYTC and WDM USA include pavement friction data, crash data, highway information systems (HIS), and resurfacing data. These datasets were combined into one central location through a combination of Microsoft Excel and Geographic Information Systems (GIS). Upon combining the datasets, the sites were efficiently prioritized based on highest risk locations to set up a desktop review and maximize KYTC's traffic safety return on investment. The datasets combined are shown in Figure 2 and described in further detail throughout the data subtopics below.
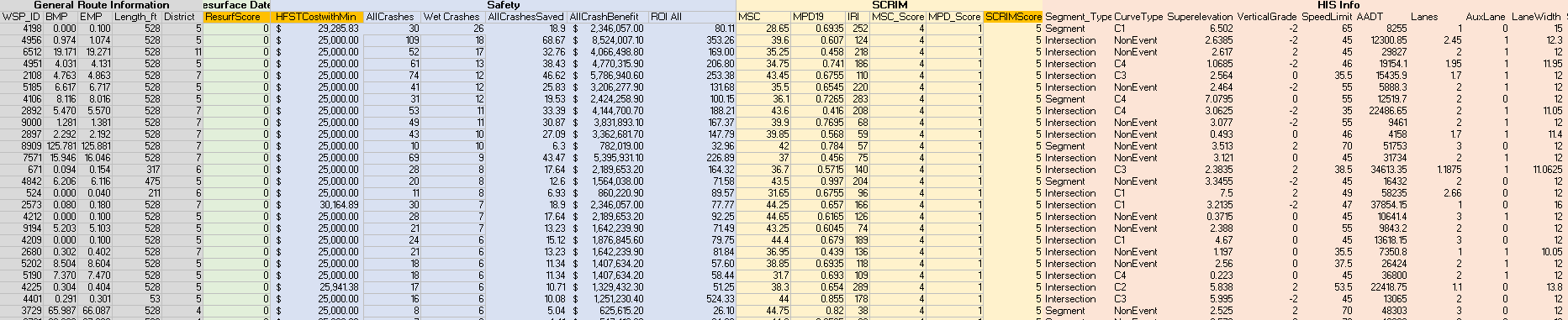
Pavement friction data
Pavement friction data is collected by WDM USA via the Sideway-force Coefficient Routine Investigation Machine (SCRIM, 2024) vehicle each year throughout the state. The SCRIM machine is shown in Figure 3. For major and divided highways, friction data is collected in both directions each year, while minor highways are collected in an alternating basis with the cardinal direction one year and the non-cardinal direction the next year. WDM USA geolocates the data by multiline string data and references to KYTC route unique, known as RT_Unique. The data are provided in 26.4-foot increments, which are aggregated into longer segments for analysis. The pavement friction data collected includes, but is not limited to:
-
Mean SCRIM Coefficient (MSC) (SCRIM, 2024),
-
Mean Profile Depth (MPD),
-
International Roughness Index (IRI),
-
Horizontal and Vertical Geometric Curvature Data,
-
Forward-facing video,
-
Global Positioning System (GPS).
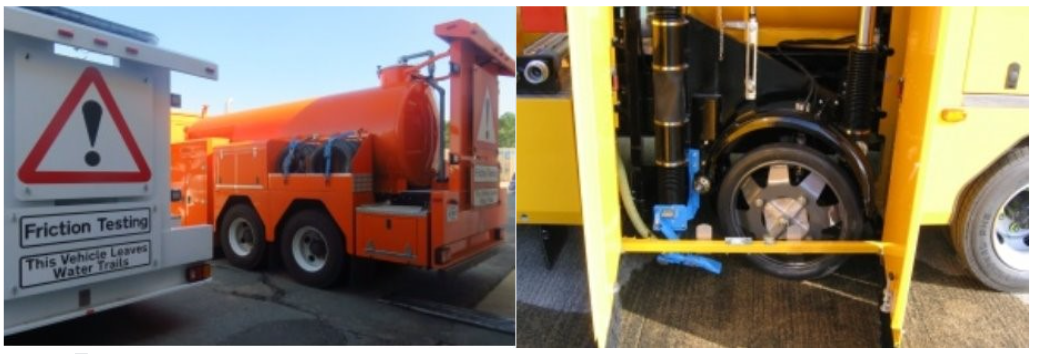
The MSC is a measure of the microtexture of the pavement surface and MPD is a measurement of macrotexture of the pavement surface. Before this study, KYTC has not endorsed particular values. To identify a process for site review and potential risk, the team used MSC and MPD ranges to create a score for this study, which are shown in Table 1. A higher score was assigned to segments with a lower MSC and MPD measurement and utilized for field visit prioritization. The MSC and MPD scores were then added together to provide a scoring metric for the desktop review, where a ‘5’ would be ranked as a top priority for field reviews.
Measures and range |
Assigned score |
---|---|
MSC |
|
75+ |
0 |
65-75 |
1 |
55-65 |
2 |
45-55 |
3 |
<35 |
4 |
MPD |
|
0.9+ |
0 |
<0.9 |
1 |
Crash data
Crash data is collected by the Kentucky State Police (KSP) and utilized by KYTC and their partners to assist in crash prevention and analytics. The KTC data team combines the KSP crash data with the crash reports to provide more detail specific to the needs of traffic safety analysts. For this study, which would include information such as wet roadway condition, speeding indication, roadway departure factors, and crash severity. Once KTC processes the crash data, it is sent to KYTC to assist with data-driven safety analysis. KTC hosts the Crash Data Access Tool (CDAT) (Green & Ross, 2021), a tool that assists traffic safety practitioners with crash data-driven approaches to evaluate locations in further detail. CDAT was utilized to examine specific crash trends around wet and roadway departure crashes throughout this study.
The KYTC HSIP team typically uses five-year crash data to provide a five-year return on investment on projects. For the first round of this study in 2023, the project team utilized crash data from 2017-2021, as the 2022 crash data was not officially finalized yet. The WDM USA team geographically tied the crash data to the appropriate segments for initial screening and safety performance function creation. The columns added include: all crashes, fatal and suspected serious injury (KA) crashes, wet roadway indicated crashes, wet roadway indicated fatal and suspected serious injury (KA) crashes, and roadway departure crashes.
Highway Information Systems
Highway Information Systems (HIS) is an online repository hosted by KYTC for types of Kentucky specific roadway data. As pavement friction data and crash data are the drivers for this study, other factors were considered when evaluating sites and noted as supplementary data. The supplementary data includes:
-
Speed Limit
-
Lane Width
-
Number of Lanes
-
Auxiliary Lanes
-
Shoulder Width
-
Roadway Horizontal Geometry
-
Roadway Vertical Geometry
-
Intersection Indication
-
Signalized Intersection Indication
-
Limited Access Freeway Ramp Indication
-
Average Annual Daily Traffic (AADT)
-
Average Annual Daily Truck Traffic (AADT).
Speed limits were identified to indicate whether speeding can be an issue. Although the speed limit does not highlight actual speeds, it can give an indication on the roadway context and be a factor for friction related treatments due to stopping sight distance (SSD) and the potential change to SSD due to friction levels. The lane width, number of lanes, and number of auxiliary lanes provides the information required for HFST placement and cost estimation, which will be discussed further in the desktop finalization. Shoulder widths were used to identify lane departure recovery. The presence of wider shoulders can highlight locations where pavement friction levels and pavement friction related crashes are low.
Roadway horizontal geometry data includes curve class by curve degree and superelevation. Curve classes range from Class A indicating a straight roadway segment to Class F indicating a small radius and sharp curve. Superelevation rates are typically associated with design speed and curve class. If the superelevation does not match the design speed or the curve class, this could indicate an issue other than pavement friction, but can still be evaluated for HFST treatments. A more severe horizontal curve class paired with other driving factors would help identify sites for a field visit confirmation.
Roadway vertical geometry data includes vertical grade class and vertical curve segment type. Vertical grade classes range from Class A indicating a flat roadway segment to Class F indicating a steep roadway segment. Vertical curve segment types highlight locations in a sag, crest, uphill, or downhill in the cardinal direction. This information can be paired with other key factors to help identify sites for a field confirmation.
Intersection indication and signalized intersection indication were added datasets due to the results of a recent study by the Pennsylvania Department of Transportation (PennDOT) and Penn State University on HFST applications at intersections (Gayah et al., 2024). Intersections associate an increase in conflict points and potential need for unexpected stopping, a key driver for pavement friction demand. Limited access freeway ramps were identified due to the crash modification factors associated with HFST on ramps, discussed further in the desktop review.
Finally, traffic demands were utilized as factors for the need and lifespan of HFST. AADT and AADTT were used to supply supplemental data as heavier traffic volumes and truck traffic volumes can wear on pavement over time and require reapplications for HFST in shorter timeframes.
KYTC district roadway resurfacing and ongoing projects data
Resurfacing data and ongoing projects were considered as a general resurfacing job or project may bring pavement friction levels back into an appropriate range. Two years of KYTC District roadway resurfacing lists were used, including the year of the data collection (2022) and the year of this project (2023). The KYTC District roadway resurfacing data is a prioritized list for resurfacing, but not all projects in the list are guaranteed to happen due to outside factors. The locations of resurfacing lists were combined through a GIS exercise to cross reference projected resurfacing projects with the list of potential HFST sites to remove any potential HFST sites with resurfacing indication.
Like resurfacing projects, current and ongoing KYTC projects were considered as project currently under construction or planned to be under construction within two years also removed potential HFST sites as a final overlay may bring pavement friction back to appropriate levels.
Desktop review
Upon collecting and combining all the data, a desktop review was conducted to identify sites for field visit. The data was combined spatially through a GIS exercise with the pavement friction data segments being the base segmentation through route unique, begin mile point, and end mile point for location identification.
Crash modification factors (CMFs) were used to estimate the number of crashes saved by applications of HFST. The CMF for curves and signals used was 0.37, ID 10327 (Merritt et al., 2020), and the CMF for ramps was 0.21, ID 10341 (Merritt et al., 2020). The CMFs applied to the five-year crash data provided an estimate of crashes saved. Due to the CMF applying to all crash severities, the USDOT estimated crash costs to be an average of $124 130 (Harmon et al., 2018). The projected number of crashes saved was multiplied by the average crash cost to get an estimate of crashes saved. The crashes saved, or the benefit, was then divided by the estimated HFST cost for each segment to provide an estimated five-year crash return on investment (ROI).
The sites were first sorted by highest friction scores and then potential five-year crash return on investment, where ‘5’ indicates the lowest MSC and MPD range and a higher ROI would suggest a strong benefit-to-cost ratio. The top sites were checked through the desktop review to utilize Google Maps, Google Earth, and the KYTC Photo Log viewer to confirm the data and to decide whether a field visit is required. The desktop review identified some other roadway issues, such as pavement condition or drainage issues, which would reduce the benefits of HFST.
Field review
Field reviews were conducted for the top sites identified after the completion of the desktop review. Field reviews looked at both objective and subjective items, including drainage issues, pavement type and condition, vehicle speeds, hard braking events, utilizing the ‘shoe test’ for friction confirmation, and other noteworthy items not seen in the data or the desktop review.
The ‘shoe test’ is a confirmation of the pavement friction. This is completed by the field employee rubbing their shoe on dry on the shoulder or off of the wheel path, rubbing their shoe in the wheel path in the direction of travel and perpendicular to the direction of travel, and finally placing water in the wheel path to repeat rubbing their shoe in the wheel path parallel and perpendicular to the direction of travel to subjectively report on whether the friction is poor or not. Figure 4 shows the shoe test being conducted during a field visit. Upon confirmation of the shoe test, pavement condition, and other noteworthy considerations, the field employees would suggest start and end locations of HFST from the field to begin desktop finalization and site selection.
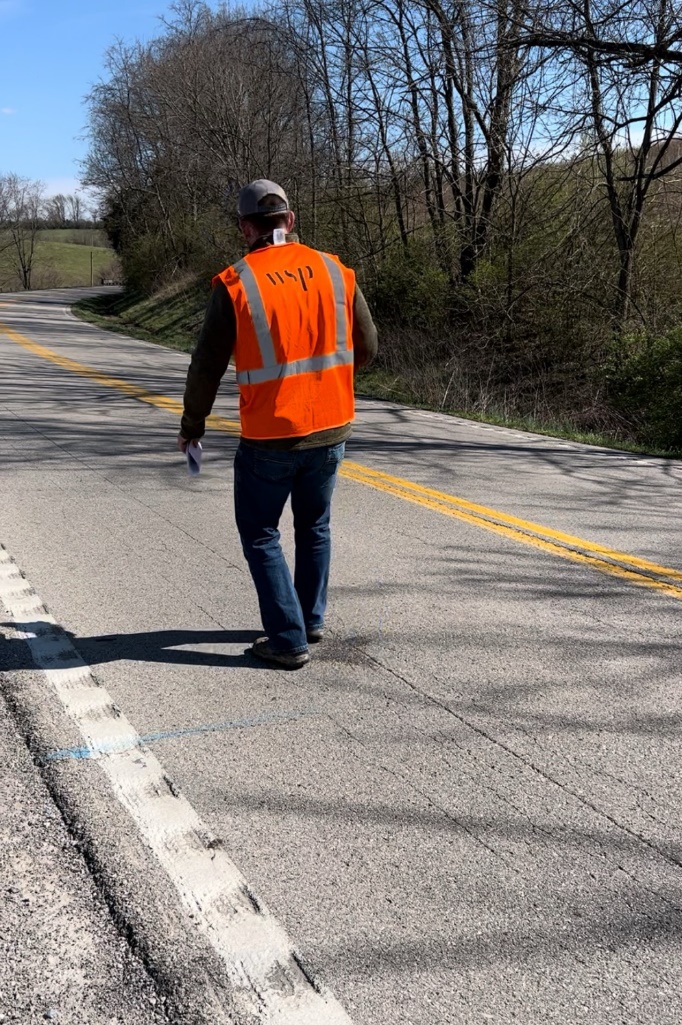
Desktop finalization
The desktop finalization brought together the final sites from the field visit and data driven process to update the segment locations for HFST placement. The desktop finalization utilized Google Earth to put the suggested bounds for HFST, recombine crash data for the new bounds, read crash narratives if applicable, estimate the cost for the site, and readjust the five-year crash ROI. Based on the adjusted data and the field visit notes, sites were rated as excellent, good, maybe, and poor candidates for HFST to prepare for a final project team review and implementation.
Formulas were used for the three different location types identified, including ramps, intersections, and segment curves. HFST on ramps started HFST one hundred feet before the curve, went through the curve, and ended one hundred feet after the curve, all between the lane markings. HFST at signalized intersections utilized AASHTO Green Book Stopping Distance calculations for the start of the HFST from the point where typical queue lengths end. Intersection HFST placement ended ten feet beyond to stop bar. Segment curves utilized information shown in Table 2 from Texas Technology Institute (Brimley & Carlson, 2012) for HFST placement:
Approach speed (mph) |
Curve speed (mph) |
||||||
---|---|---|---|---|---|---|---|
30 |
35 |
40 |
45 |
50 |
55 |
60 |
|
35 |
35 |
- |
- |
- |
- |
- |
- |
40 |
76 |
41 |
- |
- |
- |
- |
- |
45 |
122 |
86 |
46 |
- |
- |
- |
- |
50 |
173 |
138 |
97 |
51 |
- |
- |
- |
55 |
230 |
194 |
154 |
108 |
57 |
- |
- |
60 |
292 |
257 |
216 |
170 |
119 |
62 |
- |
65 |
359 |
324 |
284 |
238 |
186 |
130 |
68 |
Project team review and implementation
The final project team meeting before site selection brought together each site for the project team to decide on whether to move forward with a site for HFST application. The meeting utilized pictures and notes from the field, data collected, and priority based on the five-year ROI and cost. Upon confirmation of sites, the sites were packaged up and sent out for contract bids. To reduce costs and mobilization, sites were grouped in bidding by location proximation and site type.
Analysis and results
Before and after crash data is not ready to be completed at this time due to the recent construction of projects or projects not constructed at this time. The analysis and results of the HFST application process highlights a top-down approach of how sites were selected from initial data acquisition to final processing. Table 3 shows the two rounds of HFST and how the sites are filtered down by each phase.
Filter type |
Spring 2023 |
Fall 2023 |
---|---|---|
Initial sites |
10 000 |
5 000 |
Sites after resurfacing projects removed |
4 436 |
1 529 |
Sites listed as pavement friction score ‘5’ |
884 |
425 |
Sites for field visit |
105 |
115 |
Sites for final review |
52 |
45 |
Sites for construction letting |
41 |
34 |
The initial sites submitted to KYTC and WSP from WDM USA counted at 10 000 in spring of 2023 and 5 000 in fall of 2023. Both lists were filtered down through the data filtering process. The resurfacing projected reduced the number of sites by 55–65%. Sites listed as a pavement friction score of ‘5’, meaning lowest MSC and MPD values, reduced the list another 70–80%. Eighty sites were originally planned for a field visit, but due to proximity to other locations, the field visit added more sites for evaluation. After the field visit, around 50% of the sites were discarded due to updated crash data that does not indicate pavement friction as an issue, poor pavement condition, drainage/ponding, or engineering judgement. Finally, some sites were removed during the final review meeting as resurfacing projects may change, the five-year crash ROI was not reasonable, or another project is planned for a segment that is not outlined in the available datasets.
The estimated crashes saved for the first round is 797 worth around $98 Million and an ROI ranging from two to 106. The estimated crashes saved for the first round is 605 worth around $75 Million with an ROI ranging from three to 126. The sites constructed are being tracked for future before/after crash data metrics and official ROI.
Discussion
The data-driven process for HFST application for highway safety has shown to be an effective way of finding locations to help reduce and/or prevent fatal and serious injury, wet pavement, and roadway departure related crashes. The data process is continually being updated to create a pavement friction program within KYTC that actively contructs locations with HFST as a proven safety countermeasure. Lessons were learned with the data and process to help make the program efficient and accurate.
Lessons learned
At the beginning of the study, data mile points were not accurate to the location, but the geolocation was. The process was fixed by the next round and tied to KYTC's roadway GIS data appropriately. Due to the learning curve, the WDM USA model is able to be limited to less sites for final processing, hence why the data went from 10 000 sites to 5 000 sites between the two rounds.
The KYTC District resurfacing data was not initially understood. The data presented a list of corridors, but only certain corridors would become projects during the construction season. The KYTC Districts began providing a more detailed list of projects most likely to happen, and if the projects are not planned for the upcoming year, the chances are high for the following year.
Pavement condition became an issue on some sites. During the field visits, pictures were taken to show the pavement condition to the full project team. The field visit teams were analyzing pavement condition for excess cracking, potholes, and rutting. Some locations passed during the field visit, but between the field visit and project construction, pavement condition deteriorated. Traffic counts and truck traffic counts were added to the evaluation metrics for further supplemental data as this may impact pavement condition and lifespan of HFST projects.
Post-construction had some issues, specifically after snow events. One location had a pothole, most likely due to a mixture of pavement condition, snow/ice, and snow plows due to higher friction. This lesson learned is still under evaluation across all constructed sites.
Project implementation
The three different treatment location types included freeway ramps, signalized intersections, and segment curves. HFST along freeway ramps have been constructed over time through other projects due to the high results along similar projects. HFST placed at signalized intersections is newer and aims to have positive results in the future. Segment curves have been used in locations as a countermeasure. Some locations in this study were placed with lower five-year crash ROI's, specifically at segment curves in rural locations. These locations had a correlation of fatal and suspected serious injury crashes, paired with evidence of roadway departures upon the field visit. Although the five-year crash ROI is lower, this is due to the crash costs associated with all crashes and not specific to fatal and suspected serious injury crashes, which typically have a higher crash cost. This project was accepted for implementation to be proactive and studied. An example of a roadway segment curve with HFST application is shown in Figure 5.
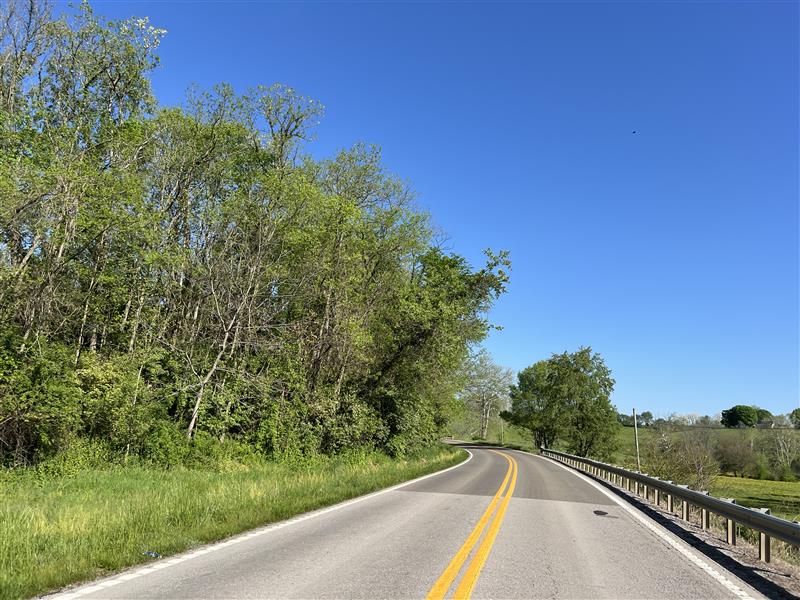
Other treatments
HFST has a high ROI for safety, but it is still a higher cost than alternate treatments and is usually placed along shorter roadway segments. Other pavement friction or texturing treatments exist and are being evaluated by the project team for future development into the Kentucky pavement texturing program. These results aim to be reported upon completion as an add on to the HFST program.
Conclusions
The Kentucky pavement friction program is continuing to research and develop to help prevent fatal and suspected serious injury crashes. The program is using a data-driven process to locate spots for HFST application specifically centered around traffic safety, typically identified through wet roadways crashes, roadway departure crashes, or higher speed locations where a major project may cost significantly more or take longer to design and develop. This project starts with robust data collection to pinpoint locations where friction may be an issue and supplementing those locations with crash data and roadway geometric data that filter down to locations for implementation. Over 70 HFST sites have been constructed or planned to be constructed throughout 2023 and 2024 through the program, while more sites are being reviewed for future development. Other pavement texturing methods are being evaluated for potential implementation as another source of pavement friction adjustments centered around traffic safety. Over time, a before and after research study will be able to complement previous research on the effects of HFST on all, wet, roadway departure crashes, and signalized intersection related crashes, potentially creating a crash modification factor for certain roadway attributes.
Declaration of competing interests
The authors report no competing interests.
Ethics statement
The methods for data collection for this project were approved by the Kentucky Transportation Cabinet (KYTC) as of 2022.
Funding
The funding for this project was supported by the Kentucky Transportation Cabinet (KYTC) Division of Traffic Operations Traffic Safety Branch.
Declaration of generative AI use in writing
The authors declare that no generative AI was used in this work.
CRediT contribution statement
Austin Obenauf: Conceptualization, Data curation, Investigation, Methodology, Resources, Validation, Visualization, Writing—original draft, Writing—review & editing.
Mike Vaughn: Conceptualization, Funding acquisition, Investigation, Methodology, Project administration, Resources, Supervision, Validation, Writing—review & editing.
Ryland Potter: Conceptualization, Data curation, Formal analysis, Investigation, Methodology, Project administration, Resources, Software, Supervision, Visualization, Writing—original draft, Writing—review & editing.
Isaac Briskin: Conceptualization, Data curation, Formal analysis, Investigation, Methodology, Resources, Software, Validation, Visualization.