Bridging the gap: understanding the factors affecting pedestrian safety perceptions in the age of driverless vehicles
Handling editor: Reginald Souleyrette, University of Kentucky, the United States of America
Reviewers: Alberto Figueroa-Medina, University of Puerto Rico, Mayagüez Campus, the United States of America
Anonymous Reviewer, not disclosed on reviewer's request
Received: 15 June 2024; Accepted: 30 November 2024; Published: 1 January 2025
Abstract
The advent of autonomous technologies necessitates a deeper understanding of pedestrian behavior and safety in environments where pedestrians need to interact with driverless vehicles (DV). Our study explores how pedestrians perceive and react to DVs compared with Human-Driven Vehicles (HDV), focusing on objective measures such as gap acceptance (GA) and psychophysiological indicators like Electro-dermal Activity (EDA). Structured in three phases, the study comprises a preliminary questionnaire to gauge public perception, followed by immersive virtual reality (VR) simulations that mimic real-world traffic scenarios within a VR environment, and concludes with a post-experiment survey. The simulation experiment was designed to analyze pedestrian responses to varying traffic scenarios developed using DVs and HDVs, measuring EDA to assess emotional and stress responses leading to changes in the gap acceptance behavior. The study employed hypothesis testing to assess DV's impact on pedestrians' psychophysiological reactions that can lead to changes in pedestrian behavior. This study also explored the effect of education level and perception of pedestrians towards automation technology that may influence outcomes. The analysis of EDA showed higher stress levels in scenarios involving DVs measured using the Galvanic Skin Response component. This result heightened stress response may be attributed to the unpredictability and novelty of DVs. The analysis with gap acceptance (GA) time revealed significant differences in GA times across traffic scenarios. Pedestrians exhibited longer GA times with DVs than HDVs, suggesting cautious crossing behavior. Our results underscore the impact of traffic scenarios on pedestrian behavior and stress levels, highlighting the influence of driverless technology on pedestrian dynamics.
Keywords
driverless vehicles, electro-dermal activity (EDA), mixed traffic scenario, pedestrian behavior, virtual reality (VR) simulations
Introduction
The rapid development and deployment of autonomous technology marks a transformative leap in surface transportation, promising enhanced road safety and efficiency. However, as these technologies become increasingly common in urban landscapes, the interface between driverless vehicles (DV) and traditional road users, especially pedestrians, emerges as a critical area of concern. Pedestrians traditionally rely on non-verifiable cues such as eye contact, hand and body gestures to gauge the driver's yielding intentions and make crossing decisions. Such interactions are nuanced and deeply embedded in societal norms, where subtle gestures can indicate whether it's safe to cross or to wait (Bennett et al., 2001; Dey & Terken, 2017). However, the transition to DVs disrupts this traditional dynamic, as the direct human element in the driving seat in vehicle control is absent. This absence poses significant challenges to pedestrian safety and decision-making processes. Pedestrians' ability to interpret vehicle's yielding intentions without human drivers becomes less predictable, potentially increasing the risk of crashes (Dey & Terken, 2017; Rasouli & Tsotsos, 2019).
While DVs can optimize vehicle stopping time and adhere to traffic laws, the lack of human-like interaction modalities necessitates new communication between DVs and pedestrians to ensure safety (Lundgren et al., 2017). Research indicates that DVs must convey their intentions clearly and in a manner that pedestrians can instinctively understand (Rasouli & Tsotsos, 2019). These new communication methods must be integrated seamlessly into the traffic system to maintain safety and efficiency. Given the variability in pedestrian behavior influenced by age, gender, and familiarity with technology, understanding pedestrian interaction with DVs becomes even more crucial (Deb et al., 2017).
While significant progress has been made in developing autonomous technologies, the empirical research on pedestrian interactions with DVs remains limited. This gap is particularly notable in studying pedestrians' complex behaviors and safety perceptions in real-world encounters with DVs. While existing research often concentrates on the technical dimensions of DVs, such as sensor accuracy and navigation algorithms, there is a critical underrepresentation of the human factors that influence pedestrian responses (Ezzati Amini et al., 2021). This absence of empirical data is a significant oversight, particularly in mixed-traffic environments where DV and human-driven vehicles (HDVs) coexist. To bridge this gap, it is essential to undertake comprehensive empirical studies that capture real-time interactions between pedestrians and DVs. By doing so, we can craft more effective communication strategies and safety protocols that are attuned to all road users' varied needs and behaviors.
Understanding stress levels and their variations among pedestrians in interactions with DVs is crucial for multiple reasons. Recent studies highlight the use of Electro-dermal Activity (EDA) to measure variations in skin conductance, reflecting emotional states like stress and providing insights into trust levels towards automated systems (Braithwaite et al., 2013). Findings indicate that lower trust correlates with higher EDA levels, revealing significant interactions between psychological responses and trust in technology (Costa et al., 2001). GA times are a critical metric in traffic engineering that measures the time interval a pedestrian is willing to accept before crossing the street after a vehicle has passed. If pedestrians tend to wait longer before crossing in front of DVs, this could have substantial implications for signal timing design and overall traffic flow efficiency. For instance, longer GA times may necessitate adjustments in signal phases at intersections to accommodate longer pedestrian wait times and prevent pedestrian congestion, especially in urban areas with heavy traffic flows.
Moreover, changes in pedestrian behavior driven by interaction with DVs require planners and engineers to rethink current pedestrian models that inform the design of crosswalks, signaling systems, and safety measures (Palmeiro et al., 2018). These changes are essential for enhancing pedestrian safety and ensuring the smooth integration of DVs into the current traffic system. Suppose DVs inherently cause pedestrians to hesitate or alter their crossing strategies. In that case, infrastructure and signal timings must evolve accordingly to mitigate any negative impacts on traffic circulation and pedestrian throughput (Figueroa-Medina et al., 2023; Zhanguzhinova et al., 2023).
Virtual reality (VR) is a powerful tool for studying complex dynamics in a controlled, safe environment. By simulating realistic traffic scenarios and allowing researchers to manipulate variables such as vehicle type, speed, and frequency, VR simulation can provide valuable insights into pedestrian decision-making processes without the risks associated with real-world testing. Additionally, VR can simulate rare or dangerous situations that are difficult to observe safely in reality, such as close interactions between pedestrians and DVs. This capability makes VR indispensable in developing and testing new traffic technologies, allowing researchers to collect detailed data on pedestrian physiological and behavioral responses. This data can then inform more accurate pedestrian models and lead to the development of DV systems that are better attuned to human behaviors and expectations, ultimately enhancing safety and efficiency in pedestrian-vehicle interactions.
This paper explores the interactions between pedestrians and DVs, examining the transformative impact of removing human drivers from vehicle-pedestrian interactions, which traditionally rely on non-verbal communication cues. Our research investigates how pedestrian behaviors, such as GA times and electro-dermal responses measured through Galvanic Skin Response (GSR), adapt in various traffic scenarios involving DVs, HDVs, and mixed conditions. We aim to understand the decision-making processes of pedestrians, the influence of their educational backgrounds and safety perceptions, and their physiological stress levels in different traffic environments. Utilizing VR simulations, this study provides a controlled yet realistic setting to explore these interactions. By addressing gaps in current research, particularly the limited understanding of real-time pedestrian responses to DVs, this study seeks to enhance pedestrian safety and predictability in increasingly automated urban landscapes. Ultimately, our findings are intended to inform innovations that could significantly reduce pedestrian fatalities and injuries, contributing to the broader discourse on human-DV interaction. The results of this research can support the development of innovative solutions to ensure the harmonious coexistence of autonomous and non-autonomous elements within urban traffic ecosystems.
Literature review
Pedestrians typically engage in three main types of interactions with drivers at crosswalks: eye contact, body gestures, and hand waving (Kitazaki & Myhre, 2015). Depending on the driver's response, these cues influence their decision to cross the roadway immediately or wait. The decision-making about crossing a road is demanding, even with human drivers, due to dense traffic and driver attentiveness. Interactions will be more complex with DV. The concept of gap acceptance refers to how pedestrians assess gaps between themselves and oncoming vehicles before crossing the road. When making a crossing choice, pedestrians may look for gaps between their reference (crossing) point and the approaching vehicle. If they think an available gap is sufficient for them to cross the road before the next vehicle arrives at the reference point, they will likely accept that gap and begin crossing; otherwise, they will reject it. Accepted gaps in interactions with HDV rarely seem shorter than 2 seconds and are more often at least 3 seconds long to enhance safety (Chandra et al., 2014). This variability highlights the complex nature of pedestrian behaviors and the need for tailored approaches in DV programming to accommodate different pedestrian strategies.
Interactions between pedestrians and autonomous systems pose unique challenges, particularly in communication and yielding intention prediction. Pedestrians might struggle to determine whether the vehicle is stopping or not, which complicates traditional cues like eye contact or gestures (Palmeiro et al., 2018; Rezwana et al., 2023). The absence of a human driver in a vehicle complicates these interactions further, demanding new forms of communication to ensure safety and clarity in pedestrian decisions (Kitazaki & Myhre, 2015). Visual and auditory signals from DVs are crucial in communicating intentions to pedestrians (Rezwana et al., 2023). Innovations in this area include using displays, lights, and projectors to convey vehicle behaviors, such as stopping or yielding (Furuya et al., 2021; Semcon, 2024; Subramanian et al., 2024). However, the effectiveness of these signals depends heavily on public familiarity and understanding, suggesting a need for widespread education and standardization to prevent miscommunication and potential crashes (Clamann et al., 2017; Fridman et al., 2017).
The exploration of DV-pedestrian interactions has been advanced by using VR), which overcomes several limitations of real-world testing, including the high costs and time requirements of building fully functional DV prototypes and testing them under real traffic conditions, the regulatory need for a test driver in such setups, and the significant physical risks to participants involved in real-world tests. VR addresses these issues and provides excellent control over experimental conditions, allowing for the easy replication of studies and the generation of extensive behavioral data through tracking systems (Feng et al., 2020; Slater & Wilbur, 1997). In recent years, the use of VR in research has surged, particularly with the advent of VR head-mounted displays (HMDs), which offer a high level of immersion, improved ergonomics, and affordability. Notably, reactions in VR settings often mimic real-life responses, and the walking speeds observed in virtual environments align with those in the real world (Deb et al., 2017). However, discrepancies remain, such as participants' tendency to engage in riskier behaviors due to the absence of real danger, their overestimation of vehicle speeds, and their acceptance of shorter time-to-contact with approaching vehicles (Bhagavathula et al., 2018; Feldstein & Dyszak, 2020; Holländer et al., 2019). Despite these challenges, the use of VR for studying pedestrian behavior, DV safety development, and DV external communication research continues to grow, yielding insights that, while sometimes limited by technical constraints like the VR systems' narrow field of view and lower resolution, are invaluable to the field (Mahadevan et al., 2019; Stanney et al., 2003). Recent research has significantly enhanced our understanding of interactions between driverless vehicles (DVs) and pedestrians, contributing to the development of safer autonomous transportation systems (Colley et al., 2019; Nascimento et al., 2019; Schneider & Bengler, 2020).
One approach to evaluating users' trust in automated systems is by examining variations in skin conductance, a measure of the skin's ability to conduct electricity, which reflects changes controlled by the sympathetic nervous system known as Electro-dermal Activity (EDA) (Braithwaite et al., 2013; Christie, 1981). EDA fluctuations are influenced by emotional states like stress, likely due to increased sweating (Costa et al., 2001; Santarcangelo et al., 2012). Recent research has explored the relationship between trust, fear, and EDA, finding that lower levels of trust are associated with higher EDA levels (Boucsein, 2012). For instance, researchers have examined GSR in correlation with human trust levels. (Khawaji et al., 2015) found significant impacts of trust and cognitive load on the EDA of individuals using a text-chat platform. Authors found that average GSR and average GSR peak values are significantly affected by both trust and cognitive load in the text-chat environment.
Additionally, a study by (Akash et al., 2018) assessed participants' trust in a sensor detecting road obstacles through trials where they were asked to trust or distrust sensor readings. Feedback was provided to validate their trust, and EDA was measured to create a trust model, highlighting these physiological markers as effective real-time indicators of trust in automation (Akash et al., 2018). Similarly, Morris et al. (2017) investigated trust and EDA responses in simulated autonomous vehicle environments during perceived risky and safe driving conditions. They reported that risky conditions led to lower trust and higher EDA among participants (Morris et al., 2017). Overall, EDA is a reliable measure of emotional arousal and provides insights into participants' emotions, actions, thoughts, and perceptions in various studies (Sequeira et al., 2009; Zhanguzhinova et al., 2023). The literature discusses using physiological measures like EDA to gauge pedestrians' emotional responses and trust levels when interacting with DVs and HDVs. These measures are crucial for assessing the psychological impacts of introducing driverless vehicles into urban traffic systems and designing interventions that enhance pedestrian trust and safety in increasingly automated environments.
In reviewing the existing literature, several vital insights emerge that are directly relevant to our study. First, traditional pedestrian-vehicle interactions rely heavily on non-verbal cues such as eye contact and gestures, which are inherently absent in interactions with DVs. This gap necessitates the development of new communication methods to ensure pedestrian safety and confidence when navigating traffic environments dominated by DVs. Previous research highlights the potential of visual and auditory signals from DVs yet underscores the need for these signals to be widely understood and standardized to be effective. Moreover, using VR simulations has proven invaluable in studying pedestrian behavior under controlled conditions. However, real-world studies remain essential to capture the full complexity of these interactions and validate VR findings.
Our study builds upon these findings by employing VR simulations to measure pedestrian responses, including gap acceptance and electro-dermal activity, across varied traffic scenarios featuring DVs, HDVs, and mixed traffic. This study also investigated how educational background and familiarity with automation influence pedestrian behavior and stress responses while interacting with DVs within a simulated environment. The study result is expected to enhance our understanding of pedestrian-DV interactions and contribute to designing safer, more intuitive communication strategies and intersection designs to significantly reduce pedestrian injuries and fatalities in urban areas.
Methodology
This study conducted a pedestrian simulation experiment in VR to understand the interactions between pedestrians and DVs while crossing a roadway. The data collection was structured into two phases: a questionnaire and a VR simulation experiment, each aimed at an in-depth analysis of the impact of DVs on pedestrian behavior and their physiological responses. The questionnaire was conducted to capture general attitudes and specific beliefs about AVs, setting a context for the experimental findings. This survey helps to understand participants' baseline perceptions and knowledge regarding autonomous systems before engaging in the VR simulation experiment. In the second step, participants were engaged with VR simulations crafted using RFpro software to emulate real-world traffic environments. The VR simulation allowed participants to walk within the VR environment as pedestrians, reacting across different traffic scenarios, including no vehicle, HDV, DV, and a mix of DVs and HDVs. The mixed traffic conditions are designed with 50% of vehicles being driverless and 50% being human-driven. Vehicles were uniformly generated and controlled, ensuring that both DVs and HDVs were evenly distributed within each trial. This uniform distribution allowed controlled comparisons between pedestrian responses to DVs and HDVs. The interaction with the pedestrians was random in mixed traffic scenarios. Concurrently, psychophysiological data such as EDA was recorded using electro-dermal sensors to assess the emotional and physiological impacts of interacting with various traffic scenarios.
A four-way signalized intersection, characterized by a two-lane major street and a one-lane minor street in each direction, was designed within a VR environment for this study, as illustrated in Figure 1. The intersection was a semi-actuated signalized intersection. The pedestrian phase was coordinated with the signal phases to ensure safe crossings. Specifically, the pedestrians were directed to cross the minor road during a dedicated pedestrian phase (protected phase) when the traffic signals on the minor road were red for vehicles, providing a safe crossing opportunity. The pedestrian phase was programmed to be activated only when a pedestrian was detected, mimicking real-world semi-actuated signal operation. During this phase, the vehicles were halted on the minor road, ensuring that pedestrians had the right of way and could cross without conflict. Additionally, the vehicles on the major road were allowed to proceed, as they did not interfere with the pedestrian crossing on the minor road. On the minor road, the lane configuration allows for shared left and right turn movements with the through lane, whereas the major road includes a dedicated left-turn lane. In this VR simulation, pedestrians are specifically directed to cross the minor street. Parking spaces are provided on both sides of the minor road, each measuring 10 feet wide and each lane 12 feet. The participants faced vehicles approaching from all travel directions while crossing the minor road. The vehicles were simulated to approach from various directions, adding complexity and realism to the scenarios.
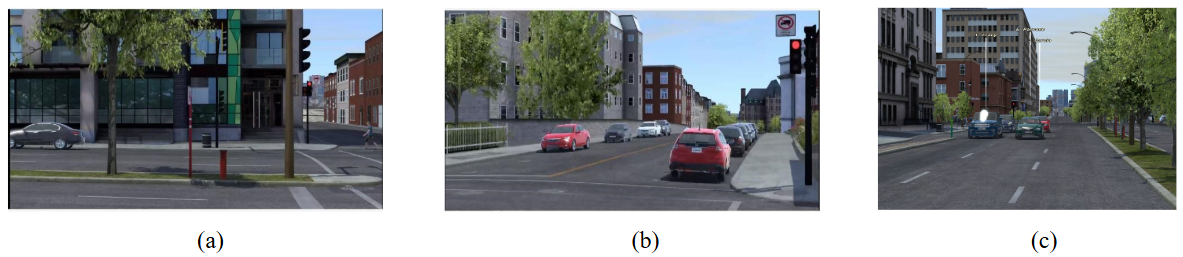
Consequently, participants in the simulation are required to navigate a crossing distance of approximately 44 feet during each trial. The scenarios are designed as 700 vehicles/hour/lane on the major road and 400 vehicles/hour/lane on the minor road in the daytime, 400 vehicles/hour/lane on the major road, and 200 vehicles/hour/lane on the minor road for nighttime. In the simulation, the turn percentages were based on realistic urban traffic patterns. Vehicles making right or left turns at the intersection were evenly distributed between the lanes, with approximately 50% of the traffic continuing with through lanes and the remaining 50% split between right (30%) and left turns (20%). The vehicle speeds were designed to be no more than 25 mph. The time headway between the vehicles was 3–4s. In this study, the behavior of both DVs and HDVs was programmed to adhere to standard traffic rules, meaning that there were no aggressive driving maneuvers, such as speeding or abrupt lane changes. The vehicles followed consistent yielding behavior, particularly at pedestrian crosswalks, to minimize variability in pedestrian responses due to driver unpredictability. This setup closely mimics realistic urban traffic conditions to assess pedestrian behavior in a controlled yet similar real-world situation.
The study consists of 7 traffic scenarios implemented within the VR environment using various traffic scenarios and lighting conditions, as presented in Table 1. The trial was defined as the event of a participant crossing the designated minor street within a simulated environment. As noted in Table 1, the participant in each scenario had to complete four trials, except the no-vehicle scenario (Scenario 1), which had only two trials. Scenario 1 was conducted at the beginning of the VR simulation experiment to familiarize the participant with the VR environment. This results in 26 trials per participant across the different scenarios. Throughout the simulation, all vehicles are programmed to adhere strictly to traffic signs, ensuring consistent conditions for each trial. Pedestrian movement is limited within the study intersection (crosswalk + sidewalk). The participants experienced each of the scenarios randomly except Scenario 1. Scenario 2 to Scenario 7 happened randomly to all the participants.
Scenarios |
Traffic scenarios |
Intersection type |
||||||
---|---|---|---|---|---|---|---|---|
No vehicle |
HDV |
Mixed traffic |
DV |
Signalized |
Day |
Night |
Trials |
|
Scenario 1 |
✓ |
✓ |
✓ |
2 |
||||
Scenario 2 |
✓ |
✓ |
✓ |
4 |
||||
Scenario 3 |
✓ |
✓ |
✓ |
4 |
||||
Scenario 4 |
✓ |
✓ |
✓ |
4 |
||||
Scenario 5 |
✓ |
✓ |
✓ |
4 |
||||
Scenario 6 |
✓ |
✓ |
✓ |
4 |
||||
Scenario 7 |
✓ |
✓ |
✓ |
4 |
The setup used to conduct the VR experiment is presented in Figure 2. The VR simulation software was integrated with Simulation of Urban Mobility (SUMO) to enhance these scenarios by managing the behavioral logic of traffic, supporting complex interactions among diverse road users. Participants used a head-mounted device in a controlled VR experiment for the immersive VR experience. GSR sensors were attached to each participant's fingers of the non-dominant hand that measured the electrical conductance, with two reusable electrodes attached to monitor the participants' physiological responses. The VR setup simulates realistic intersection scenarios with varying traffic conditions to observe pedestrian behaviors and stress levels while interacting with vehicles at crosswalks. The study involves 41 participants from the University of Connecticut and the surrounding community, who completed the study over a month. Demographic, perspective, education level, and behavioral data were collected through surveys, and VR-based responses were analyzed to study pedestrian dynamics in DV contexts.
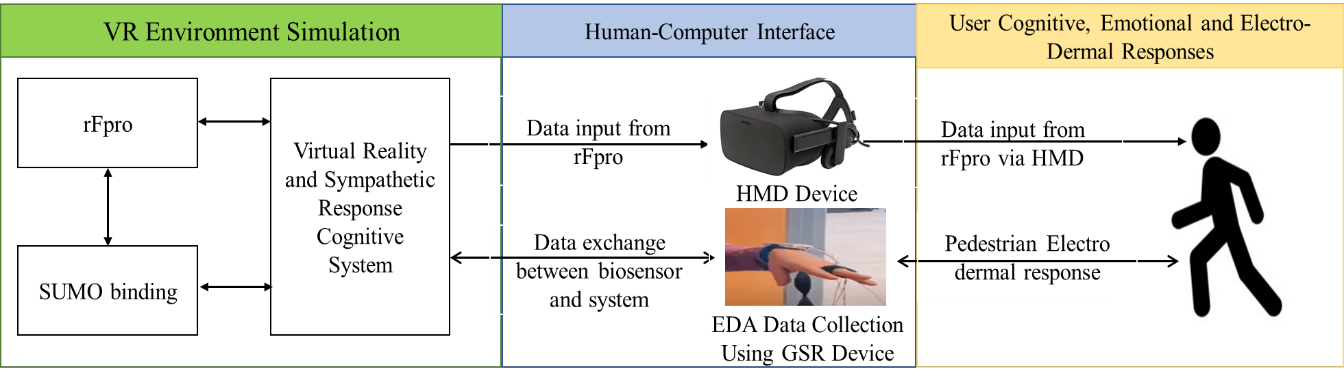
Data preparation
Before analyzing the data, we ensured the integrity and synchronization of various data sources. Video footage from GoPro cameras was aligned with VR and electro-dermal sensor timestamps, correcting any time discrepancies for accurate correlation. The complete simulation experiments resulted in 1 066 trials. The corridor where the participant walked in our VR lab, marked with reflective markers every five feet, facilitated precise tracking of pedestrian movement.
Gap acceptance was measured using RFpro screen recordings, using the formula G = Tv - Tp, where Tp is the time a pedestrian starts crossing, and Tv is when the next vehicle reaches the crosswalk. This data helped determine the safety margins pedestrians use when interacting with vehicles. The raw data from the EDA sensors, including the GSR indicator of EDA, were downloaded in CSV format and consolidated into a unified dataset. Only the GSR data was used for this study. There is potential for sudden hand movements to introduce noise into EDA measurements, particularly through pronounced peaks that are not necessarily related to the visual stimuli or events in the VR experiment. To mitigate this, noise reduction techniques were applied to the raw EDA data, including low-pass and high-pass filters, to remove artifacts and unrelated electrical noise. Furthermore, the EDA data were synchronized with video recordings to account for motion-related peaks, ensuring that the peaks considered in our analysis were more likely linked to experimental stimuli rather than extraneous movement. The GSR data underwent normalization to reduce inter-individual variability, ensuring consistent participant baseline levels.
Data analysis
Finding from questionnaire responses
The questionnaire responses demonstrated significant awareness of autonomous technology, with 97.62% of respondents acknowledging their awareness, although only 5% considered themselves highly knowledgeable. About two-thirds (66.67%) expressed positive views toward DVs, while the remainder showed skepticism or neutrality, reflecting mixed feelings about DV adoption. Safety perceptions also varied, with nearly 30% considering roadway crossings as risky, illustrating diverse attitudes towards pedestrian safety in DV contexts. The participants' educational backgrounds varied, with over 44% holding graduate or professional degrees, suggesting a highly educated sample that might influence their understanding and interactions, particularly regarding technology acceptance. Additionally, about 28% had some college education, and 18% had high school education, providing a broad range of insights into how different educational groups perceive and interact with technologies like DVs. Communication habits indicated that 67.5% of participants use non-verbal cues like hand gestures when crossing streets, underscoring the importance of visible communication for safety. Most respondents (60%) felt that technology enhances life quality, though 25% were neutral, showing some ambivalence about technology's pervasive role. The survey data provides a comprehensive view of public awareness and perceptions of DVs, showing high awareness but varying degrees of knowledge and comfort with the technology. Younger participants generally displayed more openness to DVs, while older groups were more cautiously optimistic, focusing on safety and ethical issues. These findings emphasize the need to address realistic aspects of enhancing public education on DVs and improve acceptance and confidence in this emerging technology.
Exploration of stress level and gap acceptance
The bar plot on the left illustrates the mean GSR values for the traffic scenarios. The plot shows that GSR for DVs has the highest mean GSR value, at 4.72. GSR for HDVs has a significantly lower mean GSR value of 2.58. For mixed traffic conditions, the mean GSR value is higher than the GSR level during HDV interaction but lower than the GSR level in DV traffic, at 3.49. The GSR level for the no-vehicle scenario has the lowest mean GSR value among all the traffic conditions, at 1.45. This plot provides insightful findings regarding physiological responses about the pedestrian responses towards DV. The data reveals that interactions with self-driving vehicles generate the highest GSR levels, suggesting significant arousal or stress. This heightened response is likely due to DV novelty, and perceived behavior is most likely unknown to the participant.
In contrast, scenarios involving HDVs show lower and more variable GSR levels, implying moderate stress or engagement. This variation could reflect the more predictable nature of interactions. Mixed traffic scenarios, a combination of DV and HDV, display intermediate GSR levels, the 2nd highest GSR level. The No vehicle scenario consistently shows the lowest GSR levels, indicating minimal stress or engagement and likely representing a baseline or resting state due to the absence of any vehicle in the scenario.
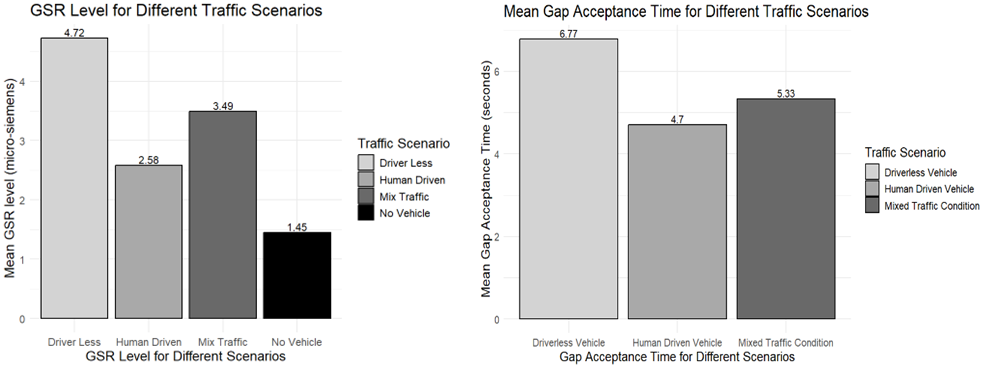
Our analysis (Figure 3, right) also examined the average GA times across three traffic scenarios—GA time for DV, GA time for HDV, and GA time for Mixed traffic. The results revealed that participants demonstrated the longest GA times in scenarios with DVs, averaging over 6 seconds, indicating a more cautious approach than in other scenarios. Conversely, GA times for HDVs averaged around 4 seconds, reflecting quicker participant decision-making. The GA times were slightly higher in mixed traffic conditions than in HDV scenarios, but they were still notable for their cautiousness.
Source |
Null hypothesis (H 0 ) |
Alternative hypothesis (H1) |
F |
p |
---|---|---|---|---|
GSR level for scenarios |
No difference in GSR levels among scenarios |
GSR levels significantly vary across different scenarios, with higher levels expected in more complex traffic conditions |
151.1 |
< 2e-16 |
Gap Acceptance (GA) time for scenarios |
No difference in Gap Acceptance (GA) times among scenarios |
GA times significantly differ across traffic scenarios, with longer GA times expected in scenarios perceived as riskier or more complex |
7.176 |
0.001 |
The ANOVA results in Table 2 demonstrate significant differences in pedestrian responses under various traffic conditions, analyzed through GA times and GSR levels. Regarding GSR levels, the data presented an even more striking difference, with an F-value of 151.1 and a p-value less than 2e-16. This outcome robustly supports the alternative hypothesis, indicating significant differences in the emotional arousal or stress levels among traffic scenarios. The highest GSR levels were observed in DV scenarios, suggesting that these scenarios induce higher stress or engagement levels among participants. This elevated response could be attributed to the unpredictability and newness of interacting with autonomous technology compared to more familiar and predictable HDV scenarios. These insights highlight the significant impact of different traffic scenarios on pedestrian behavior and physiological responses. The analysis yielded an F-value of 7.176 for GA times with a p-value of 0.001. This statistically significant result rejects the null hypothesis, affirming that different traffic scenarios influence the GA times of pedestrians. The finding suggests that pedestrians tend to be more cautious, exhibiting longer GA times, particularly in DV scenarios. This behavior reflects a heightened sense of caution, likely due to uncertainty or mistrust towards the novel autonomous technology. The results underscore the importance of considering these factors in urban planning and policymaking, particularly as cities integrate more autonomous vehicles into the traffic mix. Understanding these dynamics can help improve safety measures and pedestrian comfort in increasingly automated traffic environments.
Visual exploration of GSR and gap acceptance based on perception and education levels
A further investigation was conducted to explore the difference between GA and GSR based on the participants' perception of safety. The bar plot presented in Figure 4 provides significant insights into GA times and GSR values across different traffic scenarios—DVs, HDVs, and Mixed traffic—grouped by participants' perceptions of safety. This study explored whether DVs are perceived as creating safety issues when crossing roads. Regarding emotional arousal measured by GSR, DV data showed higher mean values for participants who perceive these vehicles as unsafe (4.84) than those who view them as safe (4.54). This increased arousal could reflect a cautious response from individuals who harbor safety concerns, potentially due to mistrust in DV safety features. For HDVs, GSR values were similar across different safety perceptions, highlighting a well-established level of familiarity and trust. Mixed traffic conditions yielded slightly higher mean GSR values for participants who viewed the situation as unsafe, suggesting that the complexity and unpredictability of these environments demand higher vigilance and engagement, especially when navigating interactions between DV and HDV.
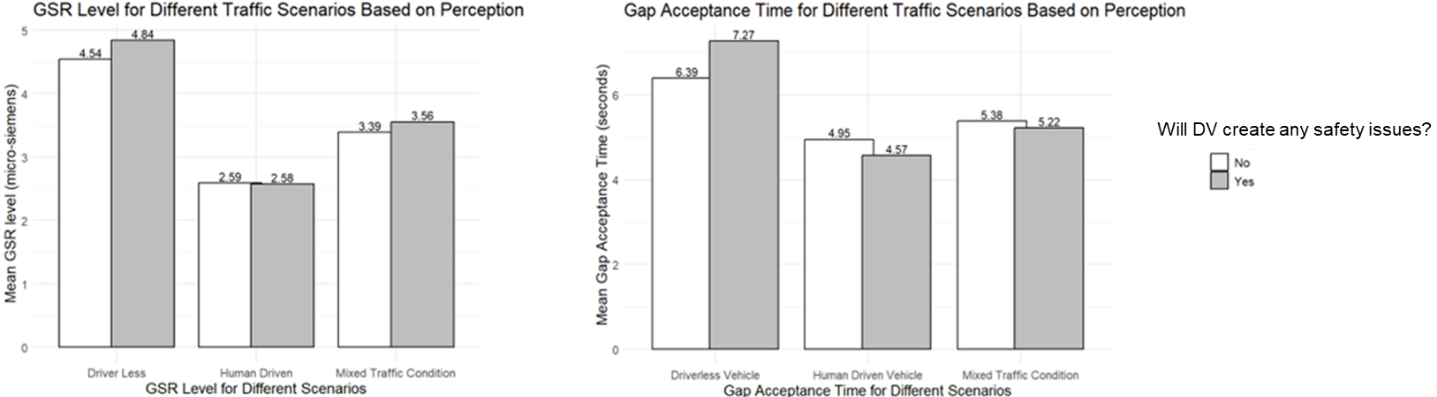
For DVs, the data reveals that participants who believe DVs will not create safety issues recorded a lower mean GA time of 6.39 seconds, compared to those who are skeptical about DV safety, demonstrating higher mean GA times of 7.27 seconds. These numbers suggest that individuals with a positive perception of DVs will likely allow smaller gaps when crossing, indicative of a higher level of trust and comfort with this technology. In contrast, GA times in HDV scenarios were relatively consistent across perceptions, indicating a uniform comfort level with traditional vehicles. The equal distribution of DV and HDV in the mixed traffic scenario may have contributed to the participants' responses. In a 50% DV and 50% HDV environment, pedestrians may rely more heavily on their familiarity with HDVs despite their concerns about DVs. As HDVs exhibit more predictable yielding behavior, participants who are more familiar with and trust HDVs might take quicker crossing actions, potentially reducing their overall GA time in mixed traffic. This effect could explain why the ‘Perception: Yes’ group—despite their safety concerns about DVs—demonstrated lower GA times than expected in mixed traffic. Mixed traffic environments may introduce an additional cognitive load due to the need to simultaneously assess two different types of vehicle behaviors. Participants concerned about DVs could be hyper-vigilant, as reflected by their higher GSR values, but they might also rely on the presence of HDVs to mitigate their hesitation. This could lead to a quicker decision to cross, particularly when they identify HDVs as part of the traffic stream, thus lowering GA times compared to a pure DV environment. These findings emphasize improving and communicating DVs' safety features to bolster public perception and acceptance. Maintaining a stable and trusted environment is crucial for HDVs. In mixed traffic scenarios, enhancing vehicle-to-vehicle communication and increasing public education on interacting with diverse traffic types could improve safety perceptions and reduce the cognitive load on pedestrians and drivers. Addressing technological advancements and public perceptions of vehicle safety is essential for fostering greater acceptance of autonomous technologies. Such efforts are vital to creating safer, more efficient roadway systems and promoting an environment that nurtures trust and comfort among all road users. This holistic approach is critical in driving broader acceptance and integration of emerging automotive technologies.
In Figure 5, The plots provided illustrate the influence of education level on GA times and GSR across different traffic scenarios, offering insights into how education may affect perception and physiological response in the context of autonomous vehicle (AV) technology. Across all traffic scenarios, high school degrees show the highest GSR levels, particularly in DV traffic conditions (4.83 micro-siemens), pointing to heightened stress in more complex traffic environments. The overall GSR level shows a decreasing value with the education level, showing that when people are more educated, they have more trust in technology. Participants with Graduate Degrees consistently show higher arousal than Doctorate holders, yet less than those with High School or Some College education, implying moderate alertness except in DV scenarios. The lowest GSR responses are observed in doctorates, especially in HDV scenarios (1.96 micro-siemens). This might reflect a more relaxed or less engaged reaction, possibly due to higher awareness of potential risks. The data suggests that lower education levels correlate with more cautious behavior and higher physiological responses in traffic scenarios involving autonomous technologies.
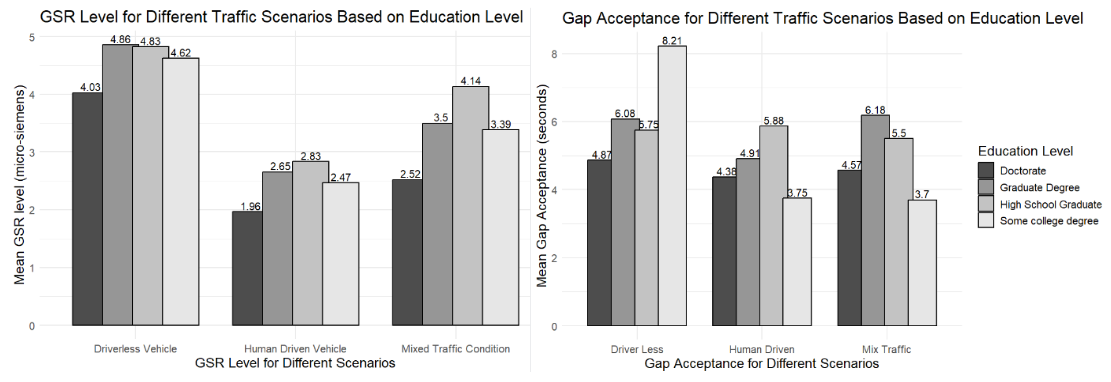
This data shows the mean GA times for DVs, HDVs, and mixed traffic conditions, segmented by participants' education levels. Notably, participants with a Doctorate exhibit the longest GA times in all scenarios, especially prominent in driverless traffic conditions (8.21 seconds), suggesting a more cautious approach, possibly due to the unfamiliarity of the complexities involved with AV technology. Those with a Graduate Degree also show longer GA times across scenarios than participants with doctorates. Interestingly, the most extended GA times are observed among participants with Some College education in DV scenarios, indicating some impact of the education level while interacting with DV. However, GA times with the participants with college degrees did not differ much for HDV and mixed traffic scenarios. This pattern could be attributed to unpredictability, potential risks, and complexities associated with DVs among less educated individuals. Conversely, those with higher education may not perceive the same level of risk, which is reflected in shorter GA times and lower GSR levels.
Figure 4; Figure 3 show a general trend where higher GSR (indicating stress or nervousness) correlates with higher GA values, reflecting more cautious crossing behavior as participants tend to take longer to cross when stressed. However, Figure 5 presents an unexpected outcome for the ‘some college degree’ group, which had the lowest GSR values across traffic conditions, suggesting lower stress and a corresponding expectation of shorter GA times. While this held for HDV and mixed traffic conditions, in the DV condition, this group exhibited the highest GA value (8.21 seconds). This suggests that, despite lower physiological stress, participants with ‘some college degree’ may have been less familiar with or trusting of DVs, leading to more cautious crossing behavior. Their low GSR may reflect comfort in the VR environment rather than trust in the DVs. This highlights the complexity of pedestrian decision-making in DV interactions, where GSR may not fully capture the cognitive and psychological factors influencing behavior.
Further research could explore the relationship between familiarity with AVs, cognitive load, and pedestrian behavior in such scenarios. This distinction highlights the need for tailored public education strategies that enhance understanding and safety practices across all education levels, ensuring that all road users can navigate new traffic technologies safely and confidently. The findings also emphasize the importance of addressing perceptual and physiological responses in integrating AV technology to enhance safety and acceptance among diverse demographic groups.
This study utilized two-way ANOVA to investigate how traffic scenarios, perceptions, and education levels influence GA times and GSR levels. In Table 3, significant differences were found in GA times among different traffic scenarios, with an F-value of 7.034 and a p-value of 0.00149, indicating that the type of traffic scenario significantly affects pedestrian decision-making. Perception also significantly influenced GA times (F = 3.053, p = 0.01907), and there was a significant interaction between traffic scenarios and perception (F = 2.604, p = 0.04878), suggesting that individual perceptions can alter the impact of traffic scenarios on GA times. For GSR levels, significant differences were observed among the traffic scenarios (F = 149.418, p < 2e-16), indicating varied emotional responses to different traffic environments. Perception significantly influenced GSR levels (F = 2.145, p = 0.0486), and the interaction between traffic scenarios and perception was also significant (F = 3.341, p = 0.0396), suggesting that how individuals perceive traffic scenarios can affect their physiological responses. When examining GSR levels based on education level, significant differences were found both among the traffic scenarios (F = 81.761, p < 2e-16) and across different education levels (F = 5.681, p = 0.00117). However, the interaction between education level and traffic scenario was not significant. For GA times based on education level, significant differences were observed among traffic scenarios (F = 7.506, p = 0.00104) and a marginal interaction effect (F = 2.023, p = 0.04226), suggesting a slight dependency on the combination of these factors. These results highlight that the type of traffic scenario and individual characteristics, such as perception and education level, significantly impact pedestrian behavior and physiological responses. This comprehensive analysis underscores the need to consider technological and perceptual aspects to enhance the safety and acceptance of autonomous vehicles.
Source |
Null hypothesis (H0) |
Alternative hypothesis (H1) |
F |
p |
---|---|---|---|---|
GSR level for traffic scenario based on perception |
||||
Traffic scenario for different GSR level |
No difference in GSR levels among scenarios |
GSR levels significantly vary across different traffic scenarios, with higher levels expected in more complex or dense traffic conditions |
149.418 |
< 2e-16 |
Perception |
No difference in GSR levels by perception |
GSR levels are significantly influenced by participants' perceptions of traffic complexity, with higher GSR levels associated with perceived higher risk scenarios |
2.145 |
0.0486 |
Interaction between GSR level and perception |
No interaction effect on GSR levels with perception |
Interaction affects GSR levels with perception |
3.341 |
0.0396 |
Gap Acceptance (GA) time for traffic scenario based on perception |
||||
Traffic scenario for different GA time |
No difference in GA times among scenarios |
GA times significantly differ across traffic scenarios, with longer GA times expected in scenarios perceived as riskier or more complex |
7.034 |
0.00149 |
Perception |
No difference in GA times by perception |
Perceptions of traffic risk or complexity significantly affect GA times, with longer times associated with higher perceived risks |
3.053 |
0.01907 |
Interaction between GA time and perception |
No interaction effect on GA times with perception |
Interaction effects GA times with perception |
2.604 |
0.04878 |
GSR level for traffic scenario based on education level |
||||
Traffic scenario for different GSR level |
No difference in GSR levels among scenarios |
GSR levels significantly vary by traffic scenarios, with distinct patterns emerging based on the educational background of participants |
81.761 |
< 2e-16 |
Education level |
No difference in GSR levels by education level |
Education level significantly influences GSR responses, with higher education levels potentially associated with lower GSR levels in complex traffic scenarios |
5.681 |
0.00117 |
Interaction between GSR and education level |
No interaction effect on GSR levels with education level |
Interaction effects GSR levels with education level |
3.442 |
0.04929 |
Gap Acceptance (GA) time for traffic scenario based on education level |
||||
Traffic scenario for different GA time |
No difference in GA times among scenarios |
Education level significantly influences GSR responses, with higher education levels potentially associated with lower GSR levels in complex traffic scenarios |
7.506 |
0.00104 |
Education level |
No difference in GA times by education level |
Education level significantly influences GA times, with higher education levels potentially associated with lower GA times in complex traffic scenarios |
3.302 |
0.02368 |
Interaction between GA time and education level |
No interaction effect on GA times with education level |
Interaction effects GA times with education level |
2.023 |
0.04226 |
Discussion
This study explores the complex interactions between pedestrians and DVs in various traffic scenarios, using VR simulations to provide a controlled yet realistic environment. The analysis of pedestrian behavior, particularly GA and GSR, offers valuable insights into how different factors, such as education level and perceptions of safety, influence pedestrian decision-making and physiological responses. The GSR data reveals higher stress levels when pedestrians interact with DVs. This increased arousal indicates that DVs are perceived as more stressful, likely due to uncertainty about the vehicles' behavior. In contrast, interactions with HDVs resulted in lower GSR levels, reflecting the familiarity and predictability of HDVs. The mixed traffic scenario elicited intermediate GSR levels, suggesting a combination of stress from the DVs and comfort from the HDVs. The findings indicate that GA data further supports these observations; pedestrians exhibit longer GA times when interacting with DVs compared to HDVs. This result of heightened caution likely stems from the novelty and unpredictability associated with DVs. In mixed traffic conditions, where DVs and HDVs are present, GA times were also longer, suggesting that DVs influence pedestrian behavior even when traditional vehicles are also on the road. This cautious approach aligns with previous studies that highlight the need for pedestrians to understand and trust the behavior of autonomous vehicles.
Significantly, the study also examined the impact of educational background and safety perceptions on pedestrian behavior. Participants with lower education levels showed higher GSR levels and higher GA times, suggesting less familiarity with and trust in DVs. Conversely, Participants with higher education levels, particularly those with doctoral degrees, displayed lower GSR levels with the shortest GA times, indicating a more analytical approach to making decisions. These individuals likely have a deeper understanding of autonomous technology's complexities and potential risks, leading to more cautious behavior. Perceptions of safety also played a crucial role. Participants who viewed DVs as safe recorded shorter GA times and lower GSR levels, indicating greater comfort and trust in the technology. Those skeptical about DVs' safety exhibited longer GA times and higher GSR levels, reflecting their apprehension and stress when interacting with autonomous vehicles.
These findings underscore the importance of public education and effective communication strategies to enhance the acceptance and safety of autonomous vehicles. Educating the public about the behavior and capabilities of DVs can help reduce stress and improve trust, leading to safer interactions. Moreover, standardizing communication methods between DVs and pedestrians, such as using clear visual and auditory signals, can help bridge the gap created by the absence of human drivers.
Conclusions
The study provides critical insights into how pedestrians interact with DVs and the factors influencing their behavior and physiological responses. The results highlight the cautious approach pedestrians take when dealing with DVs, driven by the novelty and perceived unpredictability of the technology. Education level and safety perceptions significantly impact pedestrian behavior, emphasizing the need for tailored public education and communication strategies to enhance trust and safety. As urban environments increasingly integrate autonomous vehicles, understanding these dynamics becomes essential for designing safer and more efficient traffic systems. By addressing the concerns and behaviors identified in this study, transportation authorities can develop better communication strategies and educational programs, ultimately fostering a safer coexistence between pedestrians and DVs. This research lays the groundwork for future studies to explore the interactions between pedestrians and autonomous technology further, ensuring that advancements in transportation benefit all road users.
Funding
This project was funded through The Center for Advanced Multimodal Mobility Solutions and Education (CAMMSE), a Tier 1 University Transportation Center. The Connecticut Transportation Institute and the UConn Department of Civil and Environmental Engineering also provided funding to support research facilities, staff time, and participant recruitment.
Declaration of competing interests
The authors report no competing interests.
Ethics statement
The methods for data collection in the present study have been approved by Institutional Review Board (IRB) at the University of Connecticut (Decision H22-0101).
Declaration of generative AI use in writing
The authors declare that no generative AI was used in this work.
CRediT contribution statement
Saki Rezwana: Data curation, Formal analysis, Investigation, Methodology, Visualization, Writing—original draft.
Mohammad Razaur Rahman Shaon: Conceptualization, Formal analysis, Investigation, Methodology, Project administration, Supervision, Writing—original draft, Writing—review & editing.
Nicholas Lownes: Conceptualization, Funding acquisition, Methodology, Writing—review & editing.
Eric Jackson: Conceptualization, Data curation, Project administration, Supervision, Writing—review & editing.