How does training influence use and understanding of advanced vehicle technologies: a simulator evaluation of driver behavior and mental models
Handling editor: George Yannis, National Technical University of Athens, Greece
Reviewers: Denis Kapski, Belarusian National Technical University, Belarus
Maria G. Oikonomou, National Technical University of Athens, Greece
Received: 30 June 2022; Accepted: 27 March 2023; Published: 28 March 2023
Abstract
Advanced vehicle technologies such as Advanced Driver Assistance Systems (ADAS) promise increased safety and convenience but are also sophisticated and complex. Their presence in vehicles affects how drivers interact with the technologies and how much drivers must know about these technologies. To maximize safety benefits, drivers must use such systems appropriately. They must understand how these technologies work and how they may change drivers' traditional responsibilities. Training has been recognized as an effective tool for accelerating knowledge and skills in traditional driving. Consequently, training is gaining recognition as an important tool for improving drivers' knowledge, understanding, and appropriate use of vehicle technologies as well. This study evaluated the effects of different training methods on drivers' use and understanding of vehicle automation, specifically Adaptive Cruise Control (ACC). Licensed drivers with little to no experience with ADAS features were randomly assigned into groups based on three training conditions: two experimental groups, ‘User Manual’ and ‘Visualization’, and a control group with a ‘Sham’ training. Participants were surveyed on their understanding of Adaptive Cruise Control before and after training. They also drove an advanced driving simulator equipped with ACC. The simulated drive offered multiple opportunities for the drivers to interact with the ACC and included embedded cues for engaging with the system and embedded probes to measure driver awareness of the system state. The results found a significant overall increase in knowledge of ACC after training for the experimental groups. Drivers in the experimental training groups also had better real-time awareness of the system state than the control group. The results indicate that training is associated with improved knowledge about the systems. It also shows differential effects of different approaches to training, with text-based training showing greater improvement. These findings have important implications for the design and deployment of these systems, and for policies around driver licensing and education.
Keywords
ADAS, ACC, driver training, driving simulation, mental models, vehicle automation
Introduction
Advanced vehicle technologies such as Advanced Driver Assistance Systems (ADAS) promise increased safety and convenience. These systems are being offered in most modern vehicles and thus are increasingly easily available, accessible, and achieving ubiquity (Bengler et al., 2014). However, these systems are inherently sophisticated and complex, and their presence in vehicles affects (a) how drivers interact with the technologies, and consequently (b) how much drivers must know about these technologies. These two are closely related. For the former, because the systems assist with vehicle control, a driver's role as an engaged operator changes. Relegating the control tasks to automation decreases drivers’ control responsibilities and increases monitoring responsibilities (Merat & Lee, 2012). For the latter, drivers' knowledge of system capabilities and limitations affect how appropriately they use the system. To maximize safety benefits, drivers must use the systems appropriately and correctly, and, therefore, must understand how these technologies work and how they may change drivers' traditional responsibilities. This understanding of such systems can be thought of as drivers' mental models. Carroll and Olson (1988) defined mental models as 'a rich and elaborate structure which reflects the user's understanding about the system's contents, its functionality and the concept and logic behind the functionality'.
Drivers' mental models can be influenced by design, interfaces, feedback, awareness, and training (Krampell et al., 2020). While the intuitive and careful design of ADAS can help with drivers' learning and understanding, they are complex enough that elegant design alone may not suffice, and training may be a necessary component of ADAS usage. Training has a rich history of improving driver safety and performance in traditional driving. Training has been shown to be effective in accelerating higher-order skills and knowledge in traditional driving (Pradhan et al., 2011). In a past study training was found to help calibrate trust and confidence, although in this study the improvement of knowledge and driver responses were not measured (Pai et al., 2021). However, training has an important role and is gaining recognition as a potentially critical tool for improving drivers' knowledge, understanding, and appropriate use of advanced vehicle technologies (Pradhan et al., 2019). A past simulator study found that training has helped drivers recognize edge case scenarios and take control better as compared to drivers receiving no training (Boelhouwer et al., 2019). A survey study provided different levels of information and found that the group receiving correct information had better knowledge and understanding of using ADAS (Blömacher et al., 2018). (Gaspar et al., 2020) used a similar approach in a simulator study and found that strong information led to better knowledge of ADAS. Two simulator studies with different methods of training found that any level of training helped drivers understand ADAS better, although interactive group (Forster et al., 2019) and gamified training group (Feinauer et al., 2022) demonstrated better knowledge of ADAS as compared to owner's manual. Studies have also used different methods of training such as Augmented Reality (AR) and shown that as compared to owner's manual, AR training lead to higher trust, lower ADAS interaction errors and higher user experience. Two studies by (Sportillo et al., 2018) and (Sportillo et al., 2018) used Virtual Reality (VR) as a training method and found that overall, VR method led to quick responses, and higher user experience.
Given the background and state of this field, the objective of our study was to further understand the impact of training on drivers' understanding of ADAS technologies, with a specific focus on Adaptive Cruise Control (ACC). The motivation behind the study was twofold. First, to understand the impact of training on specific technologies. Previous work has focused on a broad spectrum of technologies under the ADAS umbrella, thus the specific impact of training on any one kind of technology is not always clear. ADAS encompasses multiple types of technologies, and even if only considering, say, control-type technologies such as Adaptive Cruise Control (ACC) and Lane Keeping Assist (LKA), the two most common vehicle control type ADAS technologies, the two technologies differ vastly. Thus it is important to closely examine the impact of training content and approaches on specific technologies. Second, the study aimed to examine the role of the training approach or method in improving driver understanding.
These topics were studied using driving simulation methodologies to understand driver behavior along with surveys to understand mental models. The use of driving simulation allowed for the examination of driver responses and behaviors while they were in a more immersive driving environment where they were actually using the ADAS system. This formed a good complement to the second approach of using a mental model survey to probe drivers knowledge and understanding of these systems. This approach offers a novel way to examine training in the ADAS domain as it allows for a study of driver responses when immersed in ADAS, but without actually having to measure and understand driver interactions with any specific system. Additionally, a real-time examination of a drivers' knowledge or understanding of displays, controls, and capabilities of ADAS by using this probe paradigm may offer novel insights into driver understanding within the actual context of the task. Finally, an important contribution of this research is in the development and examination of a novel training paradigm using visualization of complex ACC systems in the form of a state diagram (as described further in Section 2.5). This approach allows for the examination of alternate methods of providing technology knowledge—without resorting to either dense and inaccessible text on one hand, or having to rely on technological approaches such as driving simulation trainers, Virtual Reality, or other complex training platforms. Given these objectives and motivations, in this study, we used a driving simulation platform to study the effects of different training methods on drivers' use and understanding of ACC. In this research, drivers' use was operationalized as the actual driver operation of systems in a simulated environment, and ‘understanding’ was assessed by measuring drivers' mental models of an ACC system.
Methodology
Objective and research questions
The objective of this study was to assess the effect of training methods on novice drivers' understanding of Adaptive Cruise Control system in a simulator study. Two experimental training methods and one control method were used to study the efficacy of training on drivers' understanding of ACC.
This research study was thus designed to answer two specific research questions:
-
Does training have a positive impact on drivers understanding of ACC?
-
What is the role of the training method in any perceived improvement in driver understanding of ACC?
Participants
Twenty-four participants were recruited to participate in the study, divided evenly by sex. The average participant age was 24.8 years (SD = 8.57 years; Minimum = 19 years; Maximum = 57 years). Participants were pre-screened for age, licensure, and understanding of ACC functionalities and limitations. Those with valid licenses with at least three months of driving experience, and between the ages of 18–65 years were eligible for the study. An important inclusion criterion was that all drivers were self-reported novice users of ADAS, i.e. with little or no knowledge of ACC.
Experimental design
A mixed, between, and within-group experimental design was used for this study, with ‘Time’ (pre-test and post-test) as the within-subject factor and ‘Training Method’ as the between-subject independent variable. The three levels of the Training Method independent variable included two experimental groups (‘User Manual’ and ‘Visualization’) and a control group (‘Sham’ training).
The dependent variables included:
-
Drivers' knowledge of ADAS as measured by a mental model survey (Completeness and Accuracy of Mental Models Survey—CAMMS, (Pradhan et al., 2022)). The survey evaluated drivers' knowledge of ACC, functionality, capabilities, and limitations. This survey was administered before training to establish a baseline knowledge of ACC and then again after training. The survey consisted of 75 unique items, and all participants answered on a scale of 1 (Strongly Disagree) to 6 (Strongly Agree). Participants' scaled agreement responses were then translated on a scale of 0 to 100. An overall Mental Models score was derived from each participant's average of all questions.
-
Accuracy of drivers' real-time verbal responses to probes about ADAS status. At various times during the drive, participants received a pre-recorded verbal probe asking questions about the state of the ACC. Participants were expected to respond to these probes verbally. Examples of these verbal probes include 'What Speed are you currently traveling at?', 'What is the current ACC Distance Setting?' or 'Is ACC currently active?'. There were six verbal probes over the duration of the drive.
-
Accuracy of drivers' real-time manual responses to instructions to operate the ADAS during the drive. During the drive, participants were instructed to perform certain operations with the ACC via a pre-recorded verbal message. The operations included actions such as changing ACC speeds or distance settings.
-
Reaction time for drivers' manual responses. Drivers' reaction times to manual responses were measured. Reaction times were measured from the completion of the instructions until the first action was taken.
Driving simulator and simulated routes
A high fidelity fixed-base full-cab driving simulator running the Realtime Technologies (RTI) SimCreator engine was used for this study. The RTI fixed-based driving simulator consists of a fully equipped 2013 Ford Fusion cab placed in front of five screens with a 330-degree field of view. The cab also features two dynamic side mirrors and a rear-view mirror which provide rear views of the scenarios for the participants. The simulator is equipped with a five-speaker surround system for exterior noise and a two-speaker system for simulating in-vehicle noise (Figure 1). RTI's SimADAS equips the simulator with ADAS features such as Adaptive Cruise Control, Traffic Jam Assist, etc. The ACC system mimics those in the real world and can maintain the vehicle's speed and distance from the lead vehicle according to the operators' set parameters. The SimCreator engine also makes it possible to script various traffic and edge case events, and introduce alerts and visual notifications to the drivers through the instrument panel and center console of the cab. In addition to vehicle measures, the simulator also collects real-time video recordings of the participants' hand movements, feet movements, and verbal responses.
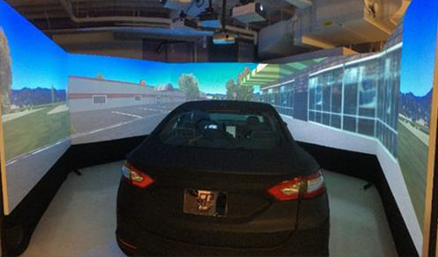
Participants were first given a five-minute training with explanation of the ACC controls (On, Off, Set Speed, Resume, etc.) and were asked to drive a short familiarization drive to help them get accustomed to the simulator.All participants drove for approximately ten minutes in the simulator. Two separate drives were designed for this experiment with a reversed sequence of driving scenarios for counterbalancing purposes. Each participant drove one of the two drives. The drives consisted of both interstate/freeway and rural/residential roadways with other traffic and road users, and commonplace driving events and scenarios. Speed limits were 65 mph on freeways and roads and 55 mph on rural roads.
Training approaches
Three training approaches were designed for this study: Visualization (V), Text-Based User Manual (M), and Sham (S).
The Visualization Training was based on prior conceptual work on advanced vehicle technologies (Pradhan et al., 2020). Accordingly, the training content included a visual representation of an ACC system as a state diagram (see Figure 2 for an example of the illustration). The diagram displayed the various possible states (or conditions) of ACC within circles. The connectors between the states explained how one could switch between states by using various controls. For example, a circle could represent an 'ACC ON' state, and another circle could represent an 'ACC OFF' state and connecting lines between the circles showed that one could move from one state to the other using the appropriate controls (i.e. the ON button on the steering wheel). The visualization was further supplemented with some instructions about limitations of ACC.
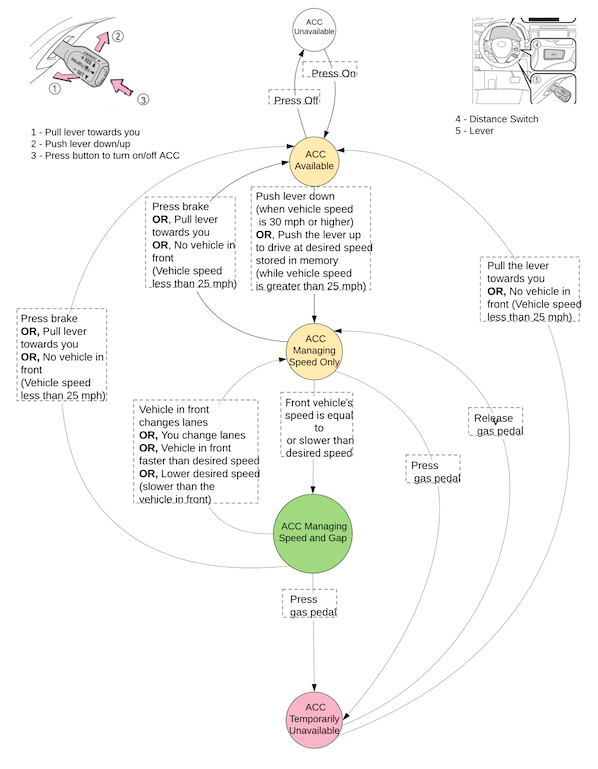
The Text-Based User Manual training included written descriptions and warnings about ACC typically found in an owner's manual. For this study, the content for this approach was compiled from actual user manuals of vehicles that offered ACC but presented in a simplified format to minimize time spent searching for relevant information. This method contained no visualizations other than schematic diagrams of control buttons.
The sham training was included in the design for a control group. This training approach consisted of text description of unrelated ADAS features, i.e. Forward Collision Warning systems (FCW) and Lane Departure Warning Systems (LDW) that were derived from user manuals and presented in a manner like the Text Based User Manual training.
Experimental procedures
The experimental study protocol was approved by the Institutional Review Board. The study required participants to visit the driving simulator laboratory. All participants completed an informed consent form. Participants then completed a demographics survey, a Trust survey (Jian et al., 2000) and the CAMMS survey (Pradhan et al., 2022). The participants were then administered the training intervention based on the condition they were randomly assigned to. Following the training, participants were again administered the trust and CAMMS survey.
After this, participants completed the simulator drives. Participants were familiarized with the driving simulator platform and the ACC system in the simulator with verbal instructions followed by a brief familiarization drive. Once participants stated they were comfortable, they started the experimental drive on the advanced driving simulator equipped with ACC. The simulated drive offered multiple opportunities for the drivers to interact with the ACC and included embedded cues for engaging with the system and embedded probes to measure driver awareness of the system state. During the drive, participants' operation of the system controls and drivers' verbal responses to embedded probes were recorded.
Data analysis
The differences between the pre-training and post-training mental models were measured through the CAMMS survey. The participants' responses were translated to a scale of 0 to 100 for correctness of the answer for all 75 items. An overall composite survey score was derived as the average of the mean. An ANOVA (analysis of variance) of the survey scores was performed across the three groups. An ANOVA is a statistical technique used to analyze variations in a response variable measured under different conditions.
Analyses were performed using R Statistical Software (v4.0.2). We compared differences in the pre- and post-training CAMMS scores individually, by using R packages such as ‘mosaic’, ‘rstatix’ and ‘ggplot to arrange and visualize individual survey items on the mental models survey across the three training groups.
Results
In this paper, we report drivers' use and understanding of ACC as measured and analyzed across the following outcomes: Knowledge of ACC, awareness of system state in real-time, and accuracy and speed of driver actions while engaging in ACC state changes.
Drivers' understanding of ACC
Figure 3 illustrates the average mental model scores (CAMMS scores) for all participants and by group. A two-way 3 (type of training method: M, S, or V) x 2 (Time Condition: Pre or Post Training Scores) mixed analysis of variance (ANOVA) with repeated measures on the survey score variable was conducted. The analysis found a significant effect of time condition but no main effect of training method. The main effect of condition type yielded an F ratio of F (1, 21) = 30.951, p < 0.001, with a significant difference between Pre-Training Survey (M = 54.255, SD = 10.32) and Post Training Survey (M = 65.455, SD = 11.83). This suggests that participants' knowledge changed between Pre and Post Training Surveys, while the training type (M, S or V) had no effect on the participants' knowledge.
The pairwise comparisons for the main effect of condition type were corrected using a Bonferroni adjustment method. The test indicates a statistically significant effect between Pre-Training and Post Training Survey, specifically for Visualization Group (p = 0.01) and Text-Based group (p = 0.04), but not for the Sham group.
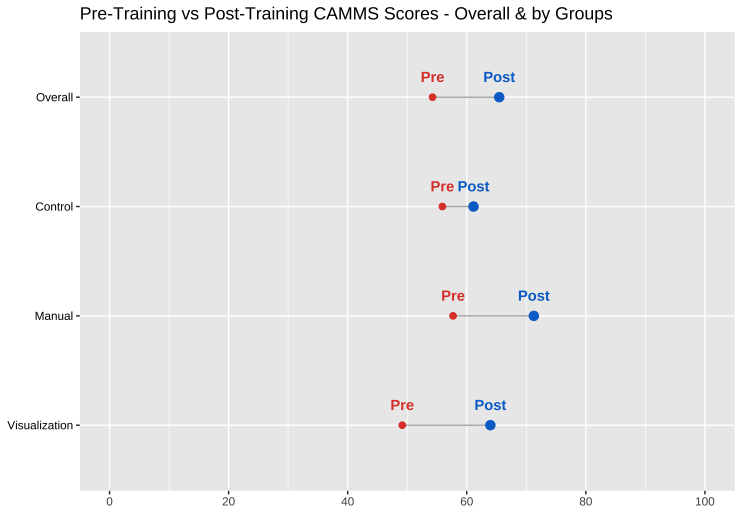
Accuracy of verbal responses
Figure 4 represents the average accuracy of the drivers' verbal responses to the probes about ACC as they drove. The participants who received user manual training and visualization training had higher mean accuracy of verbal responses (0.85 and 0.77, respectively) than the control group (0.708). A one-way ANOVA was conducted to analyze the accuracy of verbal responses based on the participants' training group. Analysis revealed that there was no main effect of the training method on the accuracy of participants' verbal responses (F = 1.4863; p = 0.229; η2 = 0.02).
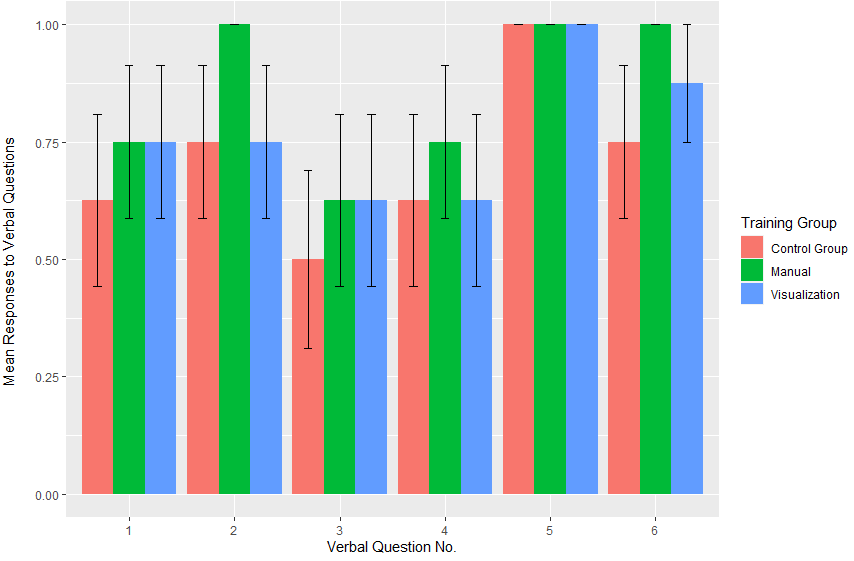
Accuracy of manual responses
The average accuracy of the drivers' manual responses to instructions is illustrated in Figure 5. Overall, the visualization group had a higher mean accuracy of manual responses (0.775) than the control group (0.75) and the manual training group (0.725). However, these differences were not statistically significant as a one-way ANOVA revealed no main effect of the training method on the accuracy of participants' manual responses (F = 0.2561; p = 0.776; η2 = 0.02).
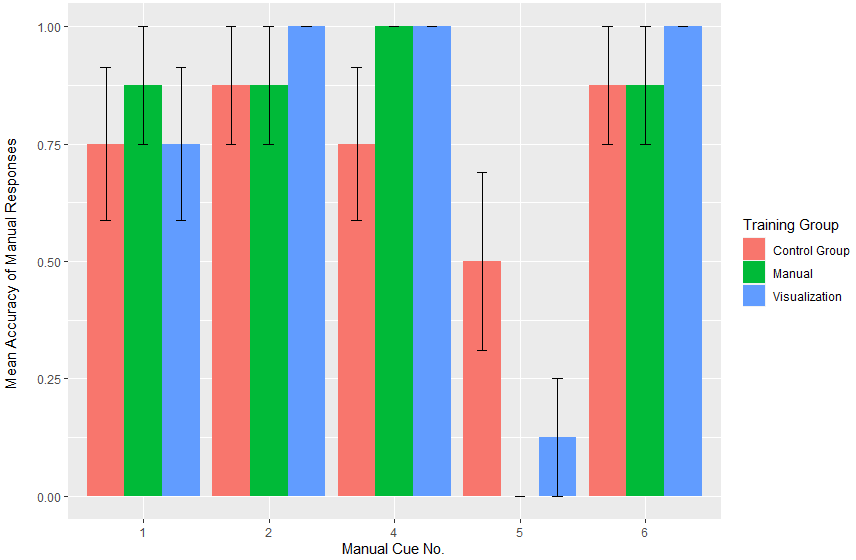
Response times for manual responses
On average, the control group took longer to manually respond (4.18 seconds) than the manual training (3.83 seconds) and visualization training (4 seconds) groups. However, a one-way ANOVA revealed that there was no main effect of the training method on the participants' response times for manual responses (F = 0.2821; p = 0.757; η2 = 0.03).
Discussion
The results of the above analyses indicate an overall increase in knowledge of ACC after training. More specifically, drivers from both the experimental training groups, i.e. those who received the Visualization training and the User Manual training, had significantly improved knowledge and understanding of ACC. This outcome underscores the potential importance of training as one significant and viable approach for improving users' knowledge of these complex vehicle systems.
The results of this study align with previous research conducted in improving driver's understanding of advanced driver assistance systems using training. Training has been known to improve knowledge of strengths and weaknesses about ADAS and improve acceptance and trust in automation (Braun et al., 2019). Reimer et al. (2010) found that before training, drivers were unaware of the benefits ADAS could provide and help in reducing stress as well as improving their performance. After training, drivers exhibited decreased stress, positive opinions and willingness to buy the system. Training has also been known to improve confidence in using and learning to use ADAS, as found by Abraham et al. (2017) in a demonstration and explanation-based study. (Koustanaï et al., 2012) provided multiple methods of training to older drivers and found that familiarization in a simulator system improved system interactions and trust in system—as compared to owner's manuals and no training. However, training of any level has been found to improve driver understanding and use of ADAS (Abraham et al., 2018).
An outcome of note from this study is that there was no significant difference in the level of improvement in knowledge between the two experimental groups. The visualization training was developed to reduce the density of information provided by user manuals and to simplify the conceptual models of ADAS with the help of illustrations of various states and ways to switch between them. User manuals are generally dense in terms of text and seem relatively inaccessible since looking for specific information about system controls means parsing through an entire user manual. There is some evidence that drivers partially or incompletely read through user manuals for their information (Mehlenbacher et al., 2002). Given these, there was a slight expectation that the visualization training may be more efficient or effective, but that was not supported by the results. One possible interpretation of this result is that the above mentioned drawbacks of a user manual may not have manifested enough in this experimental context. The user manual training consisted of excerpts from the user manual and was presented in an accessible and concise manner. Additionally, the experiment required the drivers to read the user manual excerpts. These aspects may have contributed to improved learning. In retrospect, presenting the physical user manual and requiring the users to extract the information may have been an interesting potential arm of this experimental design. In a real-world context, there may be a marked advantage of a quicker, visual method for training. This is a potential area for further extending this work to understand how drivers gain information.
When driver responses were measured in real-time and in the driving context by probing drivers during the drives, the drivers in the experimental groups generally scored more accurately than those in the control groups, but the differences were not significant. The previously discussed outcome from survey measures that drivers' knowledge was significantly better in the experimental groups after training should also have been evident in this secondary measure of knowledge, but that was not seen. A potential explanation may lie in the design of the experiment itself and, more specifically, in the content of the probes. The probed questions were relatively easy and simple, e.g. 'What is your current speed?' or 'Is ACC currently active?'. The specific probe questions used for this study may not have been sensitive enough to measure one’s deeper understanding of the system.
Similarly, the drivers' manual responses to specific system interaction instructions were more accurate for the visualization group than the user manual group or the control group, but the differences were not significant. As discussed previously, it would not be unreasonable to expect an improved manual response in the experimental drivers, given that cohort's improvement in knowledge and understanding after training. However, the current data does not support this. Again, this could be potentially explained by the fact that—just like the verbal probes—the manual instructions were relatively easy and simple (e.g. changing ACC set speed or distance). Most drivers scored at ceiling for manual performance. More complex interactions with the system may have perhaps led to more sensitivity in differentiating driver knowledge from these manual responses. Also, in terms of reaction time or speed of responses, while the experimental groups were slightly quicker at responding to probes, there was no significant difference between groups for this metric. An important learning from this study, from the perspective of empirical, experimental approaches, is that the measures chosen to study the impact on drivers' knowledge and understanding of a system are quite critical. It appears that the dependent variables chosen in this study may not have been the best or most sensitive metrics to tease out differences in drivers' understanding. This indeed returns to the original issue and the challenges in objectively measuring users' mental models. Future work is recommended in this domain, especially in ascertaining approaches to efficiently, effectively, non-invasively, and quickly measure users' knowledge of vehicle systems.
While this study indeed adds to the evidence about the importance and viability of training as a tool to improve driver knowledge, it did have some important limitations that should be considered when interpreting the outcomes. First, an important limitation is the size and makeup of the study sample. There were 24 participants, and despite aiming for a broader age range, the final participants skewed heavily towards the younger age group. While the limitation of the small sample size is obvious, it is also important to acknowledge the potential impact of a younger-skewing sample. This may have impacted the outcomes, including on direct measures such as reaction time, or indirectly by potentially influencing knowledge gain due to differences in technological familiarity or acceptance. A second important limitation is that the only system studied here was ACC. This design decision was made from an experimental control perspective with a primary goal of reducing confounds and focusing on ADAS learning rather than learning for specific flavors of ADAS. However, we recognize that ACC is vastly different from other ADAS features such as Lane Keep Assist (LKA) and even more different from the combined functionality of the two (LKA + ACC). Further exploration of the effects of training on drivers' mental models and driver behavior in the context of more complex automation is critical to better understand the use and acceptance of these technologies and to inform design and policy where the deployment of such technology is concerned. Another important future research focus would be to examine if these research outcomes generalize, not only to other types of ADAS but also to higher forms of automation and broader user populations.
Conclusions
In this study, we examined the effects of training on drivers' use and understanding of advanced vehicle technologies, namely Adaptive Cruise Control (ACC). We evaluated training approaches using a driving simulation platform. ‘Driver use’ was operationalized as actual driver operation of systems in a simulated environment, and ‘understanding’ as drivers' mental models of the system. Participants were randomly administered one of three training approaches, and the impact of training was examined by contrasting driver knowledge (mental model) before and after training and driver behaviors in terms of system use and of real-time responses about system state across three training conditions.
The study results show that training is associated with improved knowledge about the systems. It also shows the differential effects of different approaches to training, with text-based and visualization training showing more effectiveness. This data shows no significant improvement in system handling accuracy or performance after the training, indicating differences in mechanisms between understanding and using a system. Importantly, the results show that the training approach may not matter much, but that outcome may be an artifact of the experimental design and experimental context.
These findings have important implications for the design and deployment of these systems. A flawed or incomplete understanding of a system's functionalities may lead to underuse or misuse. Training and other educational approaches can help improve drivers' understanding of the systems, resulting in more appropriate and thus safer use of these systems. The results suggest that from a practical perspective, shorter and more accessible, and focused training may hold an advantage over denser, text-based user manuals, but more work is needed to understand the differences in content and delivery. The results from this study shows the promise of training and add to the relatively scarce knowledge-base on research regarding training and education for vehicle automation systems.
Declaration of competing interests
The authors report no competing interests.